
AKQ
Legend
Bronze Level
Technology and Artificial Intelligence has come to a point in which we have become beta.
The endless millions of computations and trillions of potential outcomes of all types, mean very little work to a neural network.
Some say these Neural Networks become Sentient in some way or another.
Matters not ,either way.
Meet The Greatest Poker Player In History
Say Hello to Pluribus CardsChat
https://pluribusai.com/
Now they still wont allow People inside to study Pluribus because they know its the Skynet of Poker,
But If they think that can stop Pluribus from getting on the Net ,
better think again.
Learn from the best Poker player in the world Cardschat
So I have assembled Pluribus resources to teach you all
but not by talking about it
He is just gonna do it
Lets watch
Compliments to Nino Poker
The endless millions of computations and trillions of potential outcomes of all types, mean very little work to a neural network.
Some say these Neural Networks become Sentient in some way or another.
Matters not ,either way.
Meet The Greatest Poker Player In History
Say Hello to Pluribus CardsChat
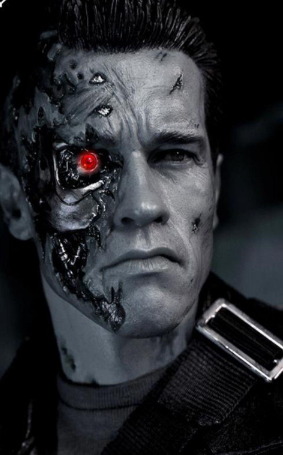
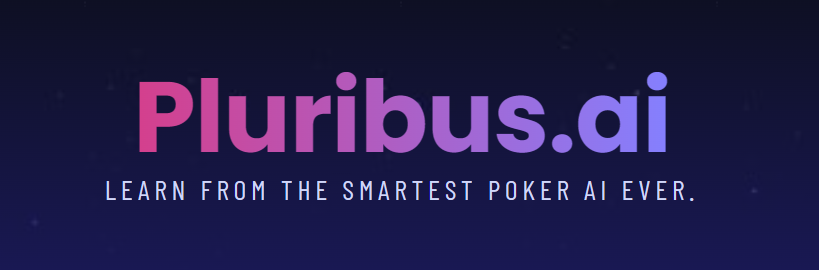
https://pluribusai.com/
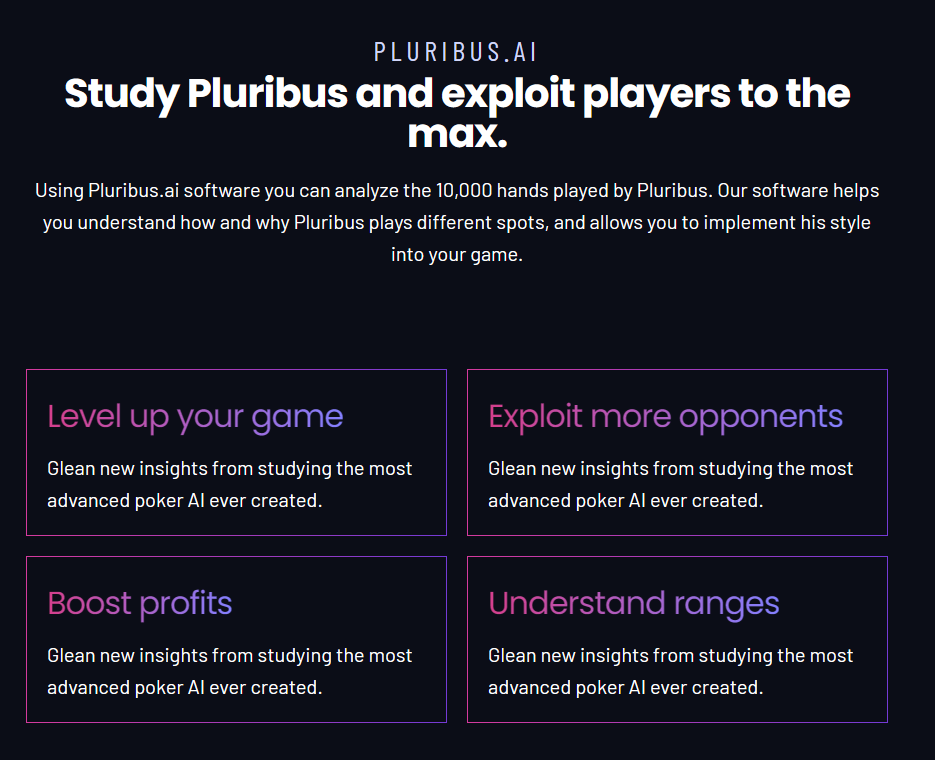
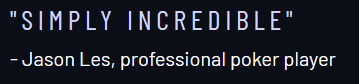
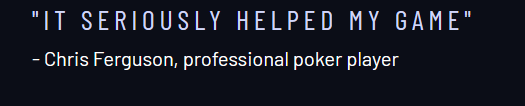
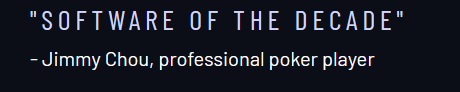
Now they still wont allow People inside to study Pluribus because they know its the Skynet of Poker,
But If they think that can stop Pluribus from getting on the Net ,
better think again.
Learn from the best Poker player in the world Cardschat
So I have assembled Pluribus resources to teach you all
but not by talking about it
He is just gonna do it
Lets watch
Compliments to Nino Poker